Complete Protection
From Financial Crimes
An end-to-end operating system that meets all your compliance requirements
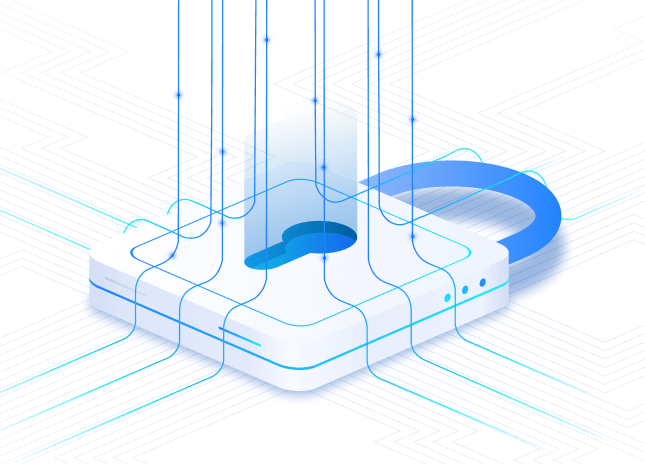
Our Impact (Annually)
5 Billion+
Transactions Monitored
400 Million+
Accounts Monitored
2 Million+
Alerts Processed
Partners Who Trust(Us
-
- |
-
- |
-
- |
-
- |
-
- |
-
- |
-
- |
-
- |
-
- |
-
- |
-
- |
-
- |
-
- |
-
- |
-
- |
-
- |
-
- |
-
- |
-
- |
-
- |
Fraud and AML Threats Are Rapidly Evolving
Rising Fraud
Risk
Growth
Introducing Tookitaki Fincense
Comprehensive | Accurate | Scalable
Stay Ahead Of Threats
Download the money laundering and transaction fraud insights shared by a global network of experts to protect against evolving fraud and money laundering scenarios.
Accurately Detect Risk In Real-Time
Automated threshold recommendations and advanced AI engine to detect suspicious patterns with 90% accuracy in real time.
Scale Seamlessly
Process billions of transactions seamlessly with a powerful modern data engineering tech stack that scales horizontally.
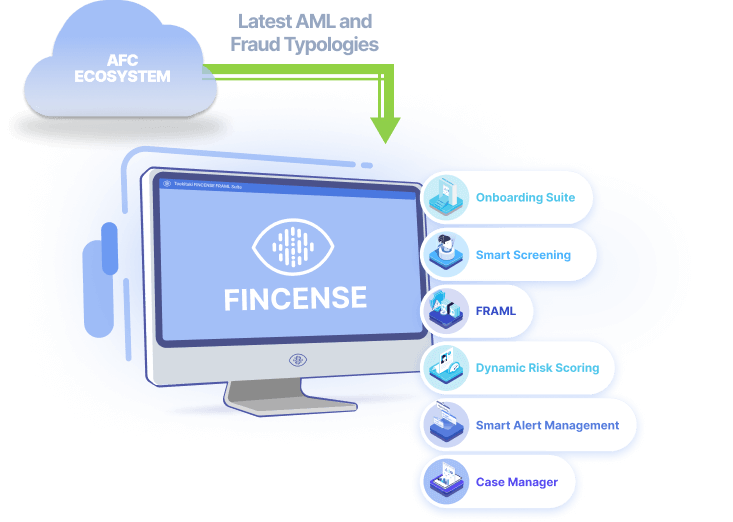
What Does this Mean For You?
100%
Risk Coverage
(Industry average: 50-60%)
90%
False Positive Reduction
(Industry average: 50%)
200
Transactions Per Second
(Industry average: 50 TPS)
Our Solutions
FRAML
Accurately prevent and detect transaction fraud and money laundering risk using automated threshold recommendations and advanced AI.
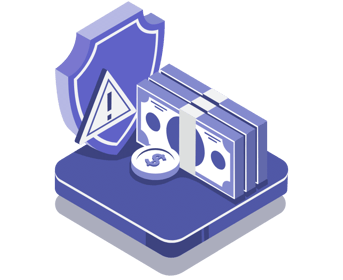
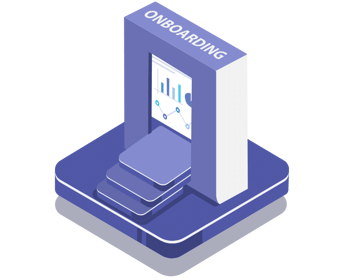
ONBOARDING SUITE
Reduce compliance friction from your onboarding process with accurate real-time screening and risk assessment of your prospects.
SMART SCREENING
Adhere to local and international regulations through accurate customer matching against sanctions, PEP, Adverse Media, and diverse watchlists in 22 languages.
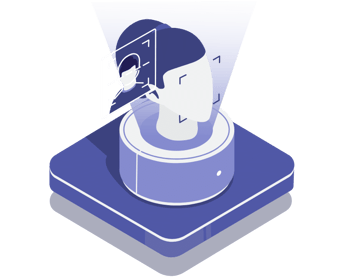
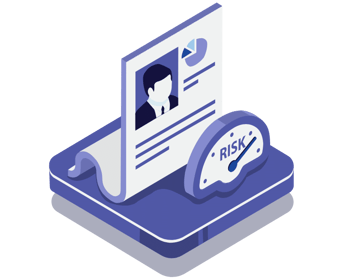
CUSTOMER RISK SCORING
Make informed business decisions using accurate customer risk profiles based on KYC, transaction, network and screening data.
SMART ALERT MANAGEMENT
Reduce false positives by up to 70% using advanced machine learning models.
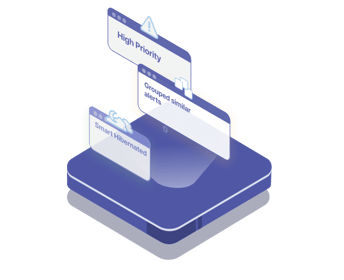
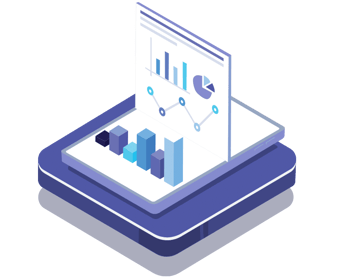
CASE MANAGER
Simplify investigations with a unified workflow and built-in automation for high efficiency.
What Do Our Customers Say?
-
Traditional Bank
-
Digital Bank
-
Payments
-
E-Wallet
Traditional Bank
Compliance Office of a Singapore Bank
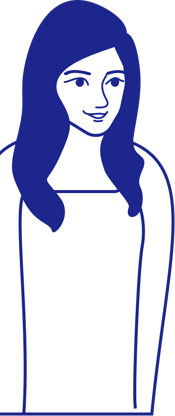
-
50%
reduction in false positives -
~45%
reduction in overall compliance cost -
Digital Bank
For a new business like ours, Tookitaki's FinCense has been a perfect partner to help us effectively manage our compliance needs.
Digital Bank Client
.png?width=220&height=416&name=Group%2014246%20(1).png)
-
100%
Risk coverage for transactions
-
50%
Reduction in time to onboard to new scenario -
Payments
FinCense's ability to detect AML and fraud risk accurately in real time allows us to maintain the performance of the system at scale. It has been a game-changer for us.
Payment Services Client
.png?width=220&height=416&name=Group%2014246%20(2).png)
-
70%
Reduction in effort on threshold tuning and scenario testing -
90%
Reduction in false positives -
E-Wallet
Tookitaki helped us simplify our compliance operations by providing us with a single platform that effectively manages all fraud and AML processes.
E-Wallet Client
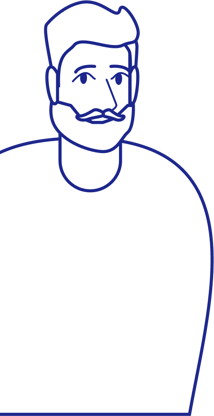
-
90%
Accuracy in high-quality alerts -
50%
Reduction in time to onboard to new scenario -
Frequently Asked Questions
Have Question? We are here to help
How can I book a demo for Tookitaki's FinCense?
You can book a demo for Tookitaki's FinCense by contacting our sales team.
What are the key features and functionalities of FinCense offered by Tookitaki?
FinCense by Tookitaki offers key features such as advanced transaction monitoring, customer risk scoring, customer due diligence, and case management.
How can FinCense help my organization in detecting and preventing money laundering activities?
The various modules of FinCense use best-in-class detection techniques that help your organization prevent money laundering activities, minimize risks and enhance compliance efforts.
Are there any specific industry regulations or compliance standards that FinCense caters to?
Yes, FinCense is developed keeping in various industry regulations and compliance standards across the globe. It can easily adapt to regulatory changes.
Can FinCense be customized to meet the specific needs and requirements of my organization?
Absolutely, FinCense can be customized to align with the specific needs and requirements of your organization.
What data sources does FinCense leverage to enhance its detection capabilities?
FinCense can integrate any number of internal and external data sources to enhance its detection capabilities.
How does FinCense handle false positives and reduce the number of false alerts?
The Smart Alert Management feature of FinCense employs advanced machine learning algorithms to reduce false positives and optimize alert accuracy.
Are there any integration capabilities with other systems or tools within my organization for seamless implementation?
Yes, FinCense offers integration capabilities with other systems or tools within your organization for a seamless implementation process.
What is the typical duration of a demo session for FinCense, and what can I expect to see during the demo?
A typical demo session for FinCense varies, and during the demo, you can expect to see live examples of its features, functionalities, and how it addresses your organization's needs.
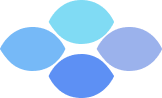
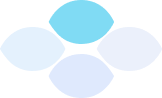
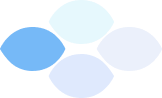
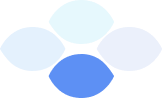
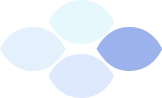
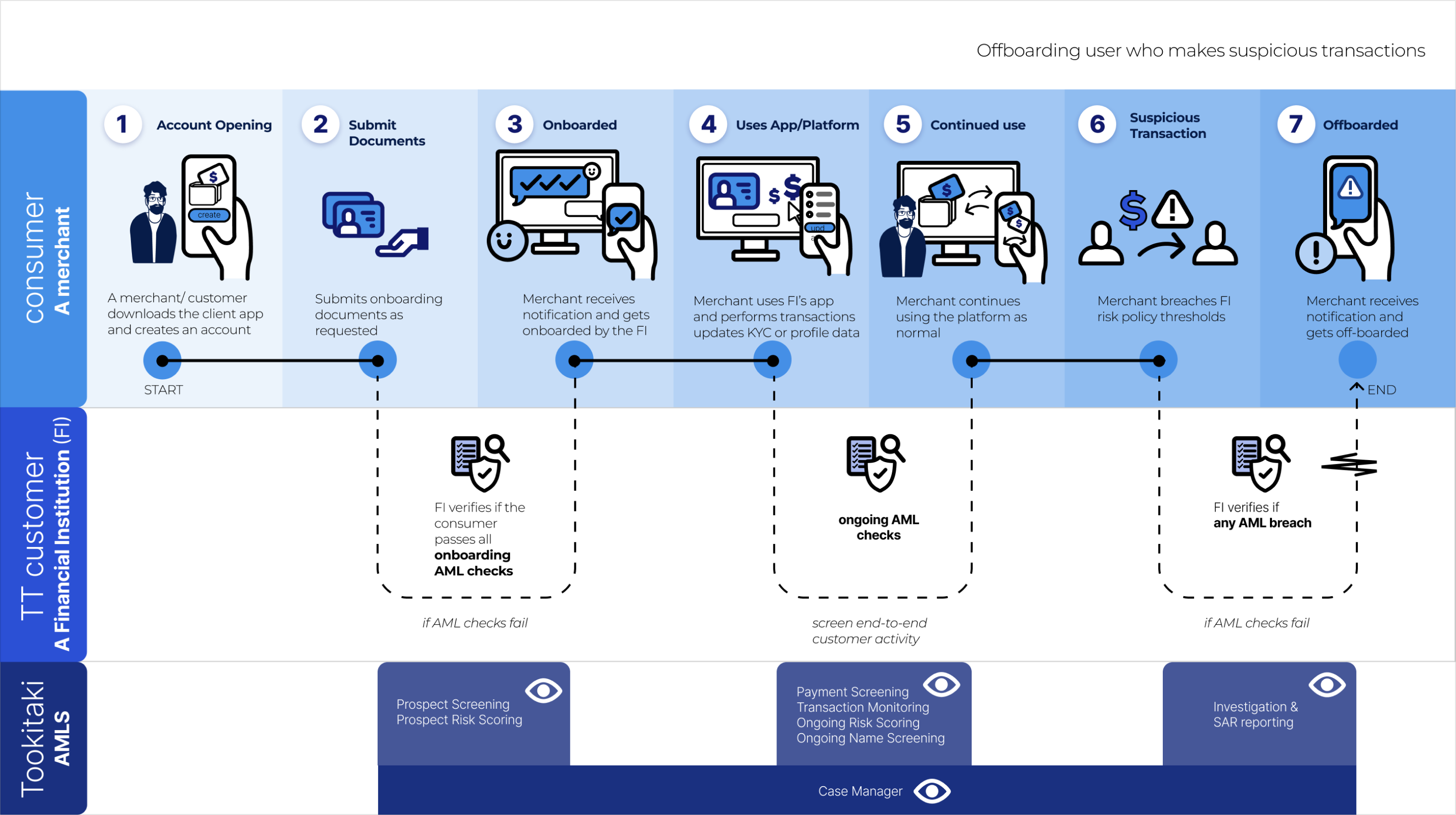
How does AMLS help you?
Scale Up Faster
The detection component of our AMLS called “Intelligent Alert Detection (IAD)” is an end-to-end suite of primary AML compliance solutions that work together seamlessly to protect your business from onboarding and ongoing AML risks without needing a large compliance team.
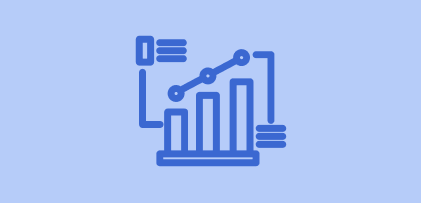
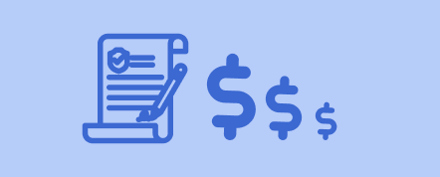
Reduce Your Compliance Cost
AMLS can also serve as a secondary AML compliance solution that will help your compliance team to sift through the large alert volumes generated by your primary AML compliance solution, identify false positives and focus on high priority risk alerts.
Our Thought Leadership Guides
Top 5 Transaction Fraud Risk Scenarios in Saudi Arabia
Top 5 Transaction Fraud Risk Scenarios in ASEAN
Navigating Screening Challenges: Part 2
Navigating AML Regulations in Singapore
Dynamic Risk Rating: The AI-Powered Future of CDD
Navigating Screening Challenges: Part 1
Choosing the Right AML Partner: Key Factors to Consider
AFC Ecosystem: Break Silos and Collaborate
Top AML Scenarios in Africa
Top AML Scenarios For Saudi Arabia
Typology Tales: Money Laundering Risks in Traditional Banks
Top Money Laundering Scenarios for ASEAN
Perpetual KYC - The Next Evolution in Risk-based Compliance
Typology Tales: High-Risk Countries and Customers
In this infographic, Tookitaki is featuring three typologies, contributed by one of our expert anti-financial crime professionals.
Typology Tales: Money Laundering Risks in Digital Banks
Prospect Screening in Real Time for Effective Risk Management
This e-book will provide an overview of prospect screening and explain the business case for real-time screening.
Automated Threshold Tuning for Optimal Alert Generation
Anti-Money Laundering (AML): A Guide for Banks and Fintechs
This e-book explores how banks and fintech companies can ensure AML compliance at a time when regulators change regulations.
Customer Due Diligence (CDD) for Banks and Fintech Companies
This guide explores the meaning of CDD, the importance of CDD, CDD methods and how financial institutions can build an effective CDD programmes.
The Paypers Financial Crime and Fraud Report 2023
The Role of Anti-Money Laundering Software in AML Compliance
This guide explores how technology can help financial institutions to manage anti-money laundering (AML) compliance.
Suspicious Activity Report (SAR): What You Need to Know
This e-book explores what constitutes a suspicious activity, who is required to file a SAR, and the SAR decision making process.
Typology Tales: Money Mules and Scams
Powering the Next-Gen of AML Technology with AI
Typology Tales: Loan Repayment
In this infographic, Tookitaki is featuring three typologies, contributed by one of our expert anti-financial crime professionals.
Money Laundering Risks in E-wallets and Digital Payments
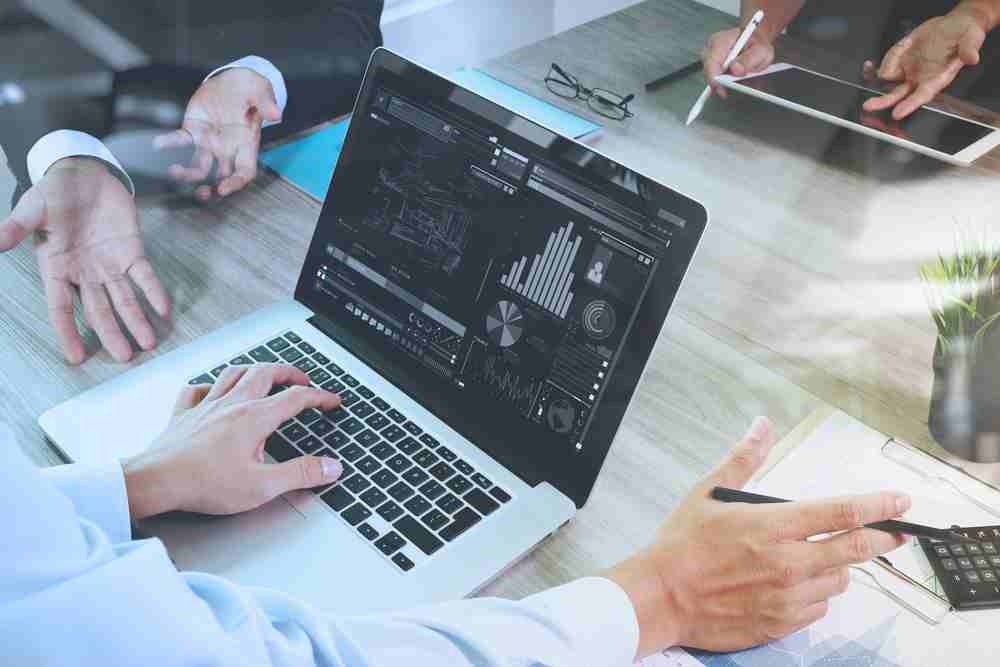